Educación
Inteligencia artificial en la educación: explicación del aprendizaje personalizado y de los conocimientos basados en datos
La inteligencia artificial está cambiando la forma en que los estudiantes aprenden, ofreciendo lecciones personalizadas y conocimientos prácticos. Profundice en el papel innovador de la IA en la educación moderna y su impacto en los resultados del aprendizaje.
Anuncios
Vea cómo la IA está convirtiendo las aulas en centros de aprendizaje inteligentes, donde las lecciones se adaptan al recorrido del estudiante.
A medida que la tecnología continúa avanzando, el sector educativo también se está adaptando para mantenerse al día con los tiempos cambiantes. Uno de los cambios más significativos en la educación es la integración de la Inteligencia Artificial (IA) en las aulas. La IA tiene el potencial de revolucionar la forma en que enseñamos y aprendemos al brindar experiencias de aprendizaje personalizadas y conocimientos basados en datos.
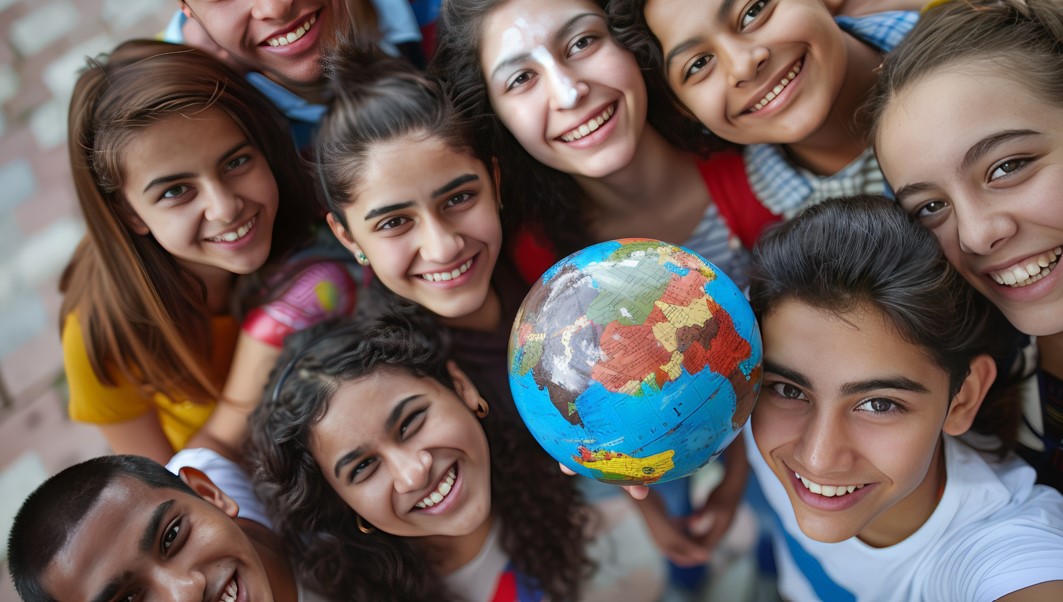
El papel de la tecnología en el intercambio cultural
Desde las redes sociales hasta la realidad virtual, explore herramientas que conectan a las personas a nivel mundial, preservando las tradiciones y fomentando la empatía y las colaboraciones innovadoras.
El aprendizaje personalizado es un enfoque que adapta la educación para satisfacer las necesidades únicas de cada estudiante. La IA desempeña un papel crucial en el aprendizaje personalizado al analizar datos de diversas fuentes, incluidas las evaluaciones, el comportamiento de los estudiantes y las preferencias de aprendizaje. Estos datos se utilizan luego para crear experiencias de aprendizaje personalizadas que se adapten a las necesidades, las capacidades y los intereses de cada estudiante. Con el aprendizaje personalizado, los estudiantes pueden progresar a su propio ritmo, lo que puede ayudar a aumentar su motivación para aprender.
La información basada en datos es otro beneficio importante de la IA en la educación. Con la IA, los educadores pueden analizar grandes cantidades de datos para obtener información sobre el rendimiento de los estudiantes, identificar áreas en las que los estudiantes pueden tener dificultades y determinar la eficacia de los métodos de enseñanza. Esta información se puede utilizar para ajustar las estrategias de enseñanza, proporcionar intervenciones específicas y mejorar los resultados de los estudiantes. Al aprovechar la IA, los educadores pueden comprender mejor las necesidades de sus estudiantes y brindar un apoyo más eficaz.
Fundamentos de la IA en la educación
La IA ha transformado el sector educativo al brindar experiencias de aprendizaje personalizadas y conocimientos basados en datos. La IA en educación se refiere al uso de algoritmos de aprendizaje automático para analizar datos de las actividades de aprendizaje de los estudiantes y brindar información sobre sus patrones de aprendizaje. Esta información se puede utilizar para personalizar la experiencia de aprendizaje de cada estudiante.
La base de la IA en la educación es el uso de datos. Los datos se pueden recopilar de diversas fuentes, como evaluaciones de estudiantes, sistemas de gestión del aprendizaje y aplicaciones educativas. Luego, estos datos se analizan mediante algoritmos de aprendizaje automático para identificar patrones y conocimientos. Estos conocimientos se pueden utilizar para personalizar la experiencia de aprendizaje de cada estudiante.
Uno de los principales beneficios de la IA en la educación es la capacidad de ofrecer experiencias de aprendizaje personalizadas. Esto significa que cada estudiante puede aprender a su propio ritmo y a su manera. Los algoritmos de IA pueden analizar los datos de las actividades de aprendizaje de un estudiante para identificar áreas en las que tiene dificultades y brindar apoyo específico. Esto puede ayudar a mejorar la participación de los estudiantes y los resultados del aprendizaje.
Otro beneficio de la IA en la educación es la capacidad de proporcionar información basada en datos. Esta información se puede utilizar para identificar áreas en las que los estudiantes tienen dificultades y proporcionar intervenciones específicas. Por ejemplo, si un estudiante tiene dificultades con un concepto en particular, el algoritmo de IA puede proporcionar recursos o actividades adicionales para ayudarlo a dominar el concepto.
En general, la IA en la educación proporciona una herramienta poderosa para mejorar los resultados de aprendizaje y brindar experiencias de aprendizaje personalizadas. Al analizar los datos de las actividades de aprendizaje de los estudiantes, los algoritmos de IA pueden brindar información sobre sus patrones de aprendizaje y brindarles apoyo específico para ayudarlos a tener éxito.
Tecnologías detrás del aprendizaje personalizado
En lo que respecta al aprendizaje personalizado, existen varias tecnologías que lo hacen posible. A continuación, se presentan tres tecnologías clave que impulsan el aprendizaje personalizado:
Sistemas de aprendizaje adaptativo
Los sistemas de aprendizaje adaptativo utilizan algoritmos de aprendizaje automático para adaptar las experiencias de aprendizaje a las necesidades individuales de los estudiantes. Estos sistemas pueden analizar los datos de rendimiento de los estudiantes y utilizar esa información para ajustar la dificultad del material de aprendizaje, proporcionar recursos adicionales u ofrecer explicaciones alternativas. Los sistemas de aprendizaje adaptativo se pueden utilizar en una variedad de entornos educativos, desde escuelas primarias y secundarias hasta instituciones de educación superior.
Análisis de aprendizaje
El análisis del aprendizaje es el proceso de recopilación y análisis de datos sobre el aprendizaje de los estudiantes para mejorar los resultados educativos. El análisis del aprendizaje se puede utilizar para identificar a los estudiantes en riesgo, realizar un seguimiento de su progreso a lo largo del tiempo y evaluar la eficacia de las intervenciones educativas. El análisis del aprendizaje también se puede utilizar para proporcionar retroalimentación en tiempo real a los estudiantes, ayudándolos a identificar áreas en las que necesitan mejorar.
Minería de datos y datos educativos
La minería de datos es el proceso de extraer información útil de grandes conjuntos de datos. En el ámbito educativo, la minería de datos se puede utilizar para identificar patrones y tendencias en los datos sobre el rendimiento de los estudiantes. Esta información se puede utilizar para desarrollar planes de aprendizaje personalizados, identificar áreas en las que los estudiantes necesitan apoyo adicional y evaluar la eficacia de las intervenciones educativas.
Los datos educativos son todos los datos que se recopilan en un entorno educativo, como datos sobre el rendimiento de los estudiantes, información demográfica e información sobre programas y recursos educativos. Estos datos se pueden utilizar para fundamentar la toma de decisiones en todos los niveles educativos, desde aulas individuales hasta distritos escolares completos. Al aprovechar los datos educativos, los educadores pueden tomar decisiones más informadas sobre cómo apoyar el aprendizaje de los estudiantes y mejorar los resultados educativos.
Estrategias de implementación
Al implementar la IA en la educación, se pueden emplear varias estrategias para garantizar una integración exitosa. Estas estrategias incluyen la integración del currículo, los roles de los docentes y los instructores y los requisitos de infraestructura.
Integración curricular
Uno de los aspectos más importantes de la implementación de la IA en la educación es la integración curricular. Esto implica incorporar herramientas y tecnologías de IA al currículo existente para mejorar la experiencia de aprendizaje. Esto se puede hacer creando nuevos cursos basados en IA o integrándola en cursos existentes. Por ejemplo, la IA se puede utilizar para personalizar las experiencias de aprendizaje al brindarles a los estudiantes contenido adaptado a sus necesidades e intereses individuales.
Roles del maestro y del instructor
Otro aspecto clave de la implementación de la IA en la educación es definir los roles de los docentes e instructores. Si bien la IA puede brindar información y apoyo valiosos, es importante recordar que no reemplaza a los docentes humanos. Los docentes e instructores seguirán desempeñando un papel importante al orientar a los estudiantes y brindar retroalimentación. La IA se puede utilizar para apoyar a los docentes brindándoles información basada en datos que puede ayudarlos a identificar áreas en las que los estudiantes pueden tener dificultades y brindar intervenciones específicas.
Requisitos de infraestructura
Por último, la implementación de la IA en la educación requiere la infraestructura adecuada. Esto incluye contar con el hardware y el software necesarios para respaldar las herramientas y tecnologías de IA. También requiere tener la infraestructura de datos adecuada para recopilar y analizar datos. Esto se puede lograr mediante la creación de un repositorio de datos centralizado que se pueda utilizar para almacenar y analizar datos de múltiples fuentes. Además, es importante garantizar que existan medidas de seguridad adecuadas para proteger los datos y la privacidad de los estudiantes.
Si sigue estas estrategias de implementación, podrá garantizar una integración exitosa de la IA en la educación. Con una implementación bien planificada, la IA puede brindar información y apoyo valiosos que pueden mejorar la experiencia de aprendizaje tanto para los estudiantes como para los docentes.
Modelos de aprendizaje personalizados
En la educación potenciada por la IA, el aprendizaje personalizado es un concepto clave que apunta a diseñar un proceso eficaz de adquisición de conocimientos que se ajuste a las fortalezas del alumno y supere sus debilidades para alcanzar en última instancia su objetivo deseado. Dos modelos populares de aprendizaje personalizado que han surgido en los últimos años son los Planes de Aprendizaje Individual (ILP, por sus siglas en inglés) y la Educación Basada en Competencias (CBE, por sus siglas en inglés).
Planes de aprendizaje individuales
Un ILP es un modelo de aprendizaje personalizado que se adapta a las necesidades e intereses específicos de cada estudiante. Es un plan integral que describe los objetivos de aprendizaje, las fortalezas, las debilidades y el estilo de aprendizaje preferido del estudiante. El ILP es creado en colaboración por el estudiante, el maestro y los padres, y se revisa y actualiza periódicamente para reflejar el progreso del estudiante y sus necesidades cambiantes.
Con la ayuda de la IA, los programas de aprendizaje integrado pueden ser aún más eficaces. Los algoritmos de aprendizaje automático pueden analizar datos de diversas fuentes, como datos de rendimiento de los estudiantes, análisis de aprendizaje y datos de comportamiento, para brindar recomendaciones personalizadas a cada estudiante. Por ejemplo, un programa de aprendizaje integrado impulsado por IA puede recomendar recursos, actividades y evaluaciones de aprendizaje específicos en función del estilo de aprendizaje, los intereses y el progreso del estudiante.
Educación basada en competencias
El CBE es un modelo de aprendizaje personalizado que se centra en el dominio de habilidades y conocimientos específicos en lugar de en la finalización de un plan de estudios establecido. En un programa CBE, los estudiantes avanzan en el plan de estudios a su propio ritmo y solo pasan al siguiente nivel una vez que han demostrado el dominio de las competencias del nivel actual.
La IA puede mejorar la educación basada en datos al brindar información basada en datos que permita a los docentes personalizar la enseñanza y el apoyo para cada estudiante. Por ejemplo, los algoritmos de aprendizaje automático pueden analizar los datos de desempeño de los estudiantes para identificar áreas en las que tienen dificultades y brindar intervenciones específicas para ayudarlos a dominar las competencias.
En conclusión, los modelos de aprendizaje personalizados, como los ILP y los CBE, son cada vez más populares en la educación potenciada por la IA. Con la ayuda de la IA, estos modelos pueden proporcionar experiencias de aprendizaje aún más personalizadas y efectivas para los estudiantes.
Perspectivas basadas en datos para la educación
A medida que la inteligencia artificial (IA) sigue transformando el sector educativo, la información basada en datos cobra cada vez mayor importancia. Al analizar los datos de los estudiantes, los educadores pueden obtener información valiosa sobre el desempeño de los estudiantes y mejorar los métodos de enseñanza. En esta sección, exploraremos dos áreas clave de información basada en datos en educación: análisis predictivo y análisis del desempeño de los estudiantes.
Análisis predictivo
El análisis predictivo es el uso de datos, algoritmos estadísticos y técnicas de aprendizaje automático para identificar la probabilidad de resultados futuros basándose en datos históricos. En educación, el análisis predictivo se puede utilizar para identificar a los estudiantes que corren el riesgo de abandonar la escuela, reprobar un curso o tener dificultades con una materia en particular.
Al analizar datos de los estudiantes, como la asistencia, las calificaciones y el comportamiento, las herramientas de análisis predictivo pueden identificar patrones y hacer predicciones sobre los resultados futuros. Esto permite a los educadores intervenir de manera temprana y brindar apoyo específico a los estudiantes que más lo necesitan.
Análisis del rendimiento estudiantil
El análisis del desempeño de los estudiantes es el proceso de analizar los datos de los estudiantes para obtener información sobre su desempeño. Al analizar los datos de los estudiantes, como las calificaciones, la asistencia y el comportamiento, los educadores pueden identificar áreas en las que los estudiantes tienen dificultades y ajustar los métodos de enseñanza en consecuencia.
Por ejemplo, si una gran cantidad de estudiantes tienen dificultades con un tema en particular, los docentes pueden ajustar sus métodos de enseñanza para abordar mejor ese tema. Además, el análisis del desempeño de los estudiantes puede ayudar a identificar a los estudiantes de alto rendimiento que pueden beneficiarse de cursos más desafiantes u oportunidades de aprendizaje acelerado.
En general, la información basada en datos es cada vez más importante en la educación. Al aprovechar el poder de la IA y las herramientas de análisis predictivo, los educadores pueden obtener información valiosa sobre el desempeño de los estudiantes y mejorar los métodos de enseñanza para satisfacer mejor sus necesidades.
Desafíos y consideraciones éticas
Como sucede con cualquier tecnología nueva, la inteligencia artificial (IA) en la educación plantea importantes desafíos y consideraciones éticas. Si bien la IA tiene el potencial de revolucionar la educación al brindar aprendizaje personalizado y conocimientos basados en datos, también plantea varias cuestiones éticas que deben abordarse.
Preocupaciones sobre la privacidad
Una de las preocupaciones éticas más urgentes en torno a la IA en la educación es la privacidad. Dado que los sistemas de IA recopilan y analizan grandes cantidades de datos sobre los estudiantes, existe el riesgo de que estos datos puedan utilizarse o manejarse de forma indebida. Es esencial garantizar que todos los datos recopilados por los sistemas de IA se mantengan confidenciales y seguros. Esto incluye la implementación de medidas sólidas de protección de datos, como el cifrado y los controles de acceso, y garantizar que los estudiantes estén completamente informados sobre cómo se utilizarán sus datos.
Sesgo y equidad
Otra preocupación ética importante es la posibilidad de que los sistemas de IA perpetúen el sesgo y la discriminación. Los sistemas de IA se basan en algoritmos que pueden estar sesgados en función de los datos con los que se entrenan. Esto significa que si los datos utilizados para entrenar un sistema de IA están sesgados, el sistema en sí estará sesgado. Para garantizar que los sistemas de IA sean justos e imparciales, es esencial utilizar conjuntos de datos diversos y representativos. Además, es crucial auditar periódicamente los sistemas de IA para identificar y abordar cualquier sesgo que pueda surgir.
Transparencia y rendición de cuentas
Por último, es necesario que los sistemas de IA que se utilizan en la educación sean transparentes y responsables. Los estudiantes, los profesores y los padres deben poder entender cómo funcionan los sistemas de IA y cómo toman decisiones sobre el aprendizaje de los estudiantes. Esto incluye ofrecer explicaciones claras sobre cómo se entrenan los sistemas de IA, qué datos utilizan y cómo llegan a sus recomendaciones. Además, es esencial establecer líneas claras de rendición de cuentas para los sistemas de IA, incluido quién es responsable de su desarrollo, mantenimiento y supervisión.
En resumen, si bien la IA en la educación tiene el potencial de transformar el aprendizaje, también plantea desafíos éticos importantes. Para garantizar que los sistemas de IA se utilicen de manera ética y responsable, es esencial abordar las preocupaciones en torno a la privacidad, los prejuicios, la equidad, la transparencia y la rendición de cuentas. De este modo, podemos aprovechar el poder de la IA para proporcionar un aprendizaje personalizado y conocimientos basados en datos, al tiempo que protegemos los derechos y la dignidad de los estudiantes.
Estudios de casos de IA en la educación
La IA se ha implementado en varias instituciones educativas y los resultados han sido prometedores. A continuación, se presentan algunos estudios de casos que muestran la eficacia de la IA en la educación:
1. Aprendizaje Carnegie
Carnegie Learning es una empresa que ofrece tutorías de matemáticas basadas en inteligencia artificial a los estudiantes. El programa utiliza tecnología de aprendizaje adaptativo para personalizar la experiencia de aprendizaje de cada estudiante. El sistema de inteligencia artificial analiza las fortalezas y debilidades del estudiante y les proporciona comentarios y recomendaciones personalizadas. En un estudio realizado por Carnegie Learning, los estudiantes que utilizaron el sistema de tutoría basado en inteligencia artificial mostraron una mejora del 63% en sus calificaciones de matemáticas en comparación con los que no utilizaron el sistema.
2. Universidad Estatal de Arizona
La Universidad Estatal de Arizona ha implementado un sistema basado en inteligencia artificial llamado eAdvisor para ayudar a los estudiantes a planificar su trayectoria académica. El sistema utiliza análisis de datos para brindar recomendaciones personalizadas a los estudiantes en función de su desempeño académico, intereses y objetivos. El sistema ha ayudado a aumentar las tasas de retención y graduación en la universidad.
3. Universidad Estatal de Georgia
La Universidad Estatal de Georgia ha implementado un sistema basado en inteligencia artificial llamado Pounce que brinda apoyo personalizado a los estudiantes que corren el riesgo de abandonar la escuela. El sistema utiliza análisis predictivos para identificar a los estudiantes que tienen dificultades académicas o económicas y les brinda apoyo y recursos personalizados. El sistema ha ayudado a aumentar las tasas de retención y graduación en la universidad.
Estos estudios de caso demuestran el potencial de la IA en la educación para brindar experiencias de aprendizaje personalizadas y conocimientos basados en datos para mejorar los resultados de los estudiantes.
Tendencias futuras en IA y educación
Tecnologías emergentes de IA
A medida que la IA siga evolucionando, se espera que tenga un impacto significativo en la educación. Una de las áreas de desarrollo más prometedoras es el aprendizaje personalizado. Los sistemas impulsados por IA pueden utilizar información basada en datos para adaptar el contenido educativo a las necesidades y capacidades de cada estudiante, lo que les permite aprender a su propio ritmo y a su manera.
Otra tecnología emergente es el procesamiento del lenguaje natural (PLN), que permite a las computadoras comprender e interpretar el lenguaje humano. Esta tecnología tiene el potencial de revolucionar la forma en que los estudiantes interactúan con el contenido educativo, haciéndolo más accesible y atractivo.
Impactos educativos a largo plazo
A largo plazo, se espera que la IA tenga un profundo impacto en la forma en que pensamos sobre la educación. A medida que los sistemas impulsados por IA se vuelvan más sofisticados, podrán brindar información cada vez más precisa y detallada sobre el desempeño de los estudiantes, lo que permitirá a los educadores identificar áreas en las que los estudiantes individuales necesitan ayuda adicional.
La IA también permitirá el desarrollo de nuevas herramientas y recursos educativos, como tutores virtuales y entornos de aprendizaje inmersivos. Estas tecnologías tienen el potencial de transformar la forma en que enseñamos y aprendemos, haciendo que la educación sea más atractiva, eficaz y accesible para todos.
En general, el futuro de la IA en la educación es prometedor y podemos esperar ver muchos avances interesantes en los próximos años. A medida que tanto los educadores como los estudiantes adopten estas nuevas tecnologías, podemos esperar un enfoque del aprendizaje más personalizado, basado en datos y eficaz.
Política y gobernanza
A la hora de implementar la IA en la educación, es esencial contar con un marco regulatorio que garantice que la tecnología se utilice de manera ética y responsable. Este marco debe incluir pautas para la recopilación de datos, la privacidad y la seguridad. También se deben considerar los posibles riesgos y beneficios de la IA en la educación y cómo mitigar los efectos negativos.
Marco regulatorio
El marco regulatorio debe diseñarse para garantizar que la IA en la educación se utilice para mejorar los resultados del aprendizaje y no para reemplazar a los docentes o reducir la calidad de la educación. También debe abordar cuestiones éticas, como el sesgo, la discriminación y la transparencia. Debe ser consciente de los riesgos potenciales de la IA en la educación, como la posibilidad de perpetuar las desigualdades existentes o crear otras nuevas.
Normas y garantía de calidad
Los estándares y la garantía de calidad son componentes fundamentales de cualquier política de IA en educación. Se deben desarrollar estándares para garantizar que los sistemas de IA sean precisos, confiables y eficaces para lograr sus propósitos previstos. Se deben establecer mecanismos de garantía de calidad para monitorear el desempeño de los sistemas de IA y garantizar que cumplan con los estándares requeridos.
En resumen, las políticas y la gobernanza son componentes esenciales de la implementación de la IA en la educación. Debe existir un marco regulatorio para garantizar que la IA se utilice de manera ética y responsable, y deben desarrollarse estándares y mecanismos de garantía de calidad para garantizar que los sistemas de IA sean precisos, confiables y eficaces para lograr los propósitos previstos.
Perspectivas de las partes interesadas
En lo que respecta a la IA en la educación, las partes interesadas tienen distintas perspectivas sobre cómo se puede implementar para mejorar el aprendizaje personalizado y la información basada en datos. A continuación, se presentan algunas perspectivas clave de estudiantes, educadores y administradores.
Estudiantes
Los estudiantes son los principales beneficiarios del aprendizaje personalizado impulsado por IA. Con IA, los estudiantes pueden recibir experiencias de aprendizaje personalizadas que se adaptan a sus necesidades y capacidades únicas. Las plataformas de aprendizaje impulsadas por IA pueden proporcionar recomendaciones personalizadas para cursos, materiales de estudio y evaluaciones. Además, los estudiantes pueden recibir comentarios en tiempo real sobre su progreso, lo que les permite ajustar sus estrategias de aprendizaje en consecuencia.
Sin embargo, algunos estudiantes pueden tener inquietudes sobre el uso de la IA en la educación. Por ejemplo, pueden preocuparse de que las evaluaciones impulsadas por IA puedan ser sesgadas o injustas. Es importante que los educadores y administradores aborden estas inquietudes y se aseguren de que la IA se utilice de una manera transparente y equitativa.
Educadores
Los educadores desempeñan un papel fundamental en la implementación del aprendizaje personalizado impulsado por la IA y la información basada en datos. La IA puede ayudar a los educadores a identificar áreas en las que los estudiantes pueden tener dificultades y brindar intervenciones específicas para ayudarlos a tener éxito. Además, la IA puede ayudar a los educadores a ahorrar tiempo en tareas administrativas, lo que les permite centrarse más en la enseñanza y la interacción con los estudiantes.
Sin embargo, algunos educadores pueden dudar en adoptar la IA en el aula. Les preocupa que pueda reemplazar la interacción humana o que no sea eficaz para satisfacer las necesidades de todos los estudiantes. Es importante que los educadores reciban capacitación sobre cómo usar la IA de manera efectiva y que participen en el proceso de toma de decisiones cuando se trata de implementar la IA en el aula.
Administradores
Los administradores son responsables de tomar decisiones sobre el uso de la IA en la educación. Deben equilibrar los posibles beneficios de la IA con las preocupaciones en torno a la privacidad, la ética y la equidad. La IA puede ayudar a los administradores a tomar decisiones basadas en datos sobre la asignación de recursos, el apoyo a los estudiantes y el desarrollo del plan de estudios.
Sin embargo, los administradores también deben garantizar que el uso de la IA sea transparente y equitativo. Deben ser transparentes en cuanto a cómo se utiliza la IA y qué datos se recopilan. Además, deben asegurarse de que la IA no perpetúe sesgos o desigualdades en el sistema educativo.
En general, las partes interesadas tienen distintas perspectivas sobre el uso de la IA en la educación. Si bien existen preocupaciones en torno a la privacidad, la ética y la equidad, la IA tiene el potencial de revolucionar el aprendizaje personalizado y la información basada en datos en la educación. Es importante que las partes interesadas trabajen juntas para garantizar que la IA se utilice de una manera que beneficie a todos los estudiantes y promueva la equidad y la transparencia en la educación.
Preguntas frecuentes
¿Cuáles son los beneficios de utilizar IA en entornos de aprendizaje personalizados?
Los entornos de aprendizaje personalizados impulsados por IA ofrecen una variedad de beneficios tanto para los estudiantes como para los educadores. Al aprovechar los algoritmos de aprendizaje automático, los sistemas de IA pueden analizar los datos de los estudiantes y brindar recomendaciones personalizadas en función de sus necesidades de aprendizaje específicas. Esto puede generar una mayor participación de los estudiantes, un mejor desempeño académico y mayores tasas de retención. Además, la IA puede ayudar a los educadores a identificar áreas en las que los estudiantes tienen dificultades y brindar intervenciones específicas para ayudarlos a tener éxito.
¿Cómo puede la IA mejorar los conocimientos basados en datos para mejorar los resultados educativos?
La IA puede ayudar a los educadores a recopilar y analizar grandes cantidades de datos de los estudiantes, como resultados de evaluaciones, niveles de participación y patrones de comportamiento. Al extraer información significativa de estos datos, los docentes pueden comprender mejor a cada estudiante y tomar decisiones informadas para personalizar sus experiencias de aprendizaje de manera eficaz. Esto puede generar mejores resultados educativos, como un aumento del rendimiento estudiantil y de las tasas de graduación.
¿De qué manera los algoritmos impulsados por IA están transformando los procesos de enseñanza y aprendizaje?
Los algoritmos impulsados por IA están transformando los procesos de enseñanza y aprendizaje de varias maneras. Por ejemplo, la IA puede ayudar a los educadores a crear rutas de aprendizaje personalizadas para los estudiantes en función de sus necesidades y preferencias individuales. Además, la IA puede proporcionar retroalimentación en tiempo real a los estudiantes, lo que les permite ajustar sus estrategias de aprendizaje y mejorar su desempeño. Por último, la IA puede ayudar a los educadores a identificar áreas en las que necesitan mejorar sus métodos de enseñanza y brindar oportunidades de desarrollo profesional específicas para ayudarlos a crecer.
¿Cuáles son algunos ejemplos exitosos de aprendizaje personalizado impulsado por inteligencia artificial?
Existen varios ejemplos exitosos de aprendizaje personalizado impulsado por inteligencia artificial. Por ejemplo, la plataforma Carnegie Learning utiliza IA para brindar instrucción matemática personalizada a los estudiantes. De manera similar, la plataforma Knewton utiliza IA para crear rutas de aprendizaje personalizadas para los estudiantes en función de sus necesidades y preferencias individuales. Por último, la plataforma DreamBox Learning utiliza IA para brindar instrucción matemática personalizada a estudiantes de jardín de infantes a octavo grado.
¿Cómo apoya la IA la individualización de las experiencias de aprendizaje en la educación superior?
La IA puede contribuir a la individualización de las experiencias de aprendizaje en la educación superior al ofrecer recomendaciones personalizadas a los estudiantes en función de sus necesidades de aprendizaje específicas. Por ejemplo, la IA puede ayudar a los estudiantes a seleccionar cursos y especializaciones que se ajusten a sus intereses y objetivos profesionales. Además, la IA puede ofrecer comentarios personalizados a los estudiantes sobre su desempeño académico, lo que les permite ajustar sus estrategias de aprendizaje y mejorar sus calificaciones.
¿Cuáles son los desafíos y las consideraciones éticas de la implementación de IA en entornos educativos?
La implementación de la IA en entornos educativos implica varios desafíos y consideraciones éticas. Por ejemplo, existe el riesgo de que los sistemas de IA refuercen los sesgos y las desigualdades existentes en el sistema educativo. Además, existe el riesgo de que los sistemas de IA infrinjan los derechos de privacidad de los estudiantes al recopilar y analizar sus datos personales sin su consentimiento. Por último, existe el riesgo de que los sistemas de IA reemplacen a los docentes humanos, lo que provocaría la pérdida de puestos de trabajo y otras consecuencias negativas. Es importante que los educadores y los responsables de las políticas aborden estos desafíos y consideraciones éticas a medida que implementan la IA en entornos educativos.
Tendencias
También te puede interesar
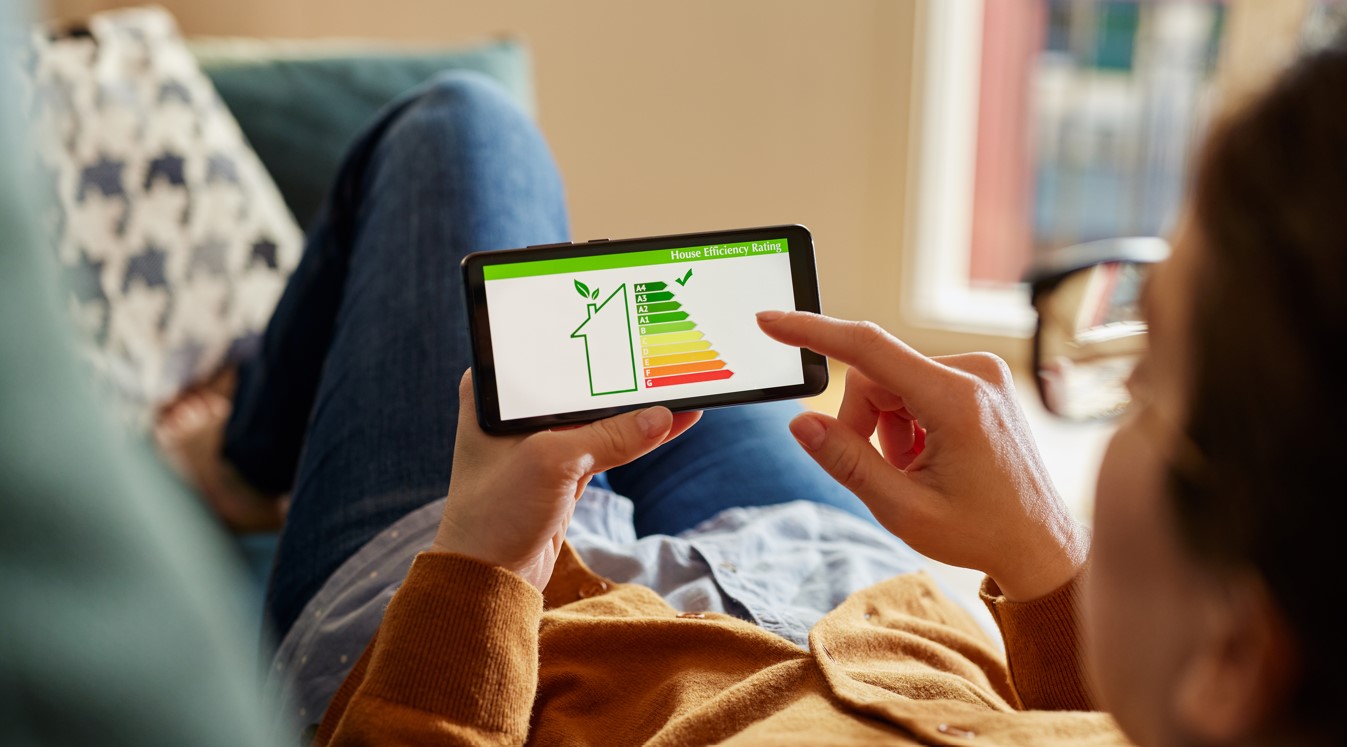
Soluciones de eficiencia energética: cómo ahorrar energía con electrodomésticos, edificios y redes inteligentes
Descubra soluciones prácticas de eficiencia energética para hogares y empresas, desde electrodomésticos inteligentes hasta edificios optimizados y redes inteligentes.
Continúe Leyendo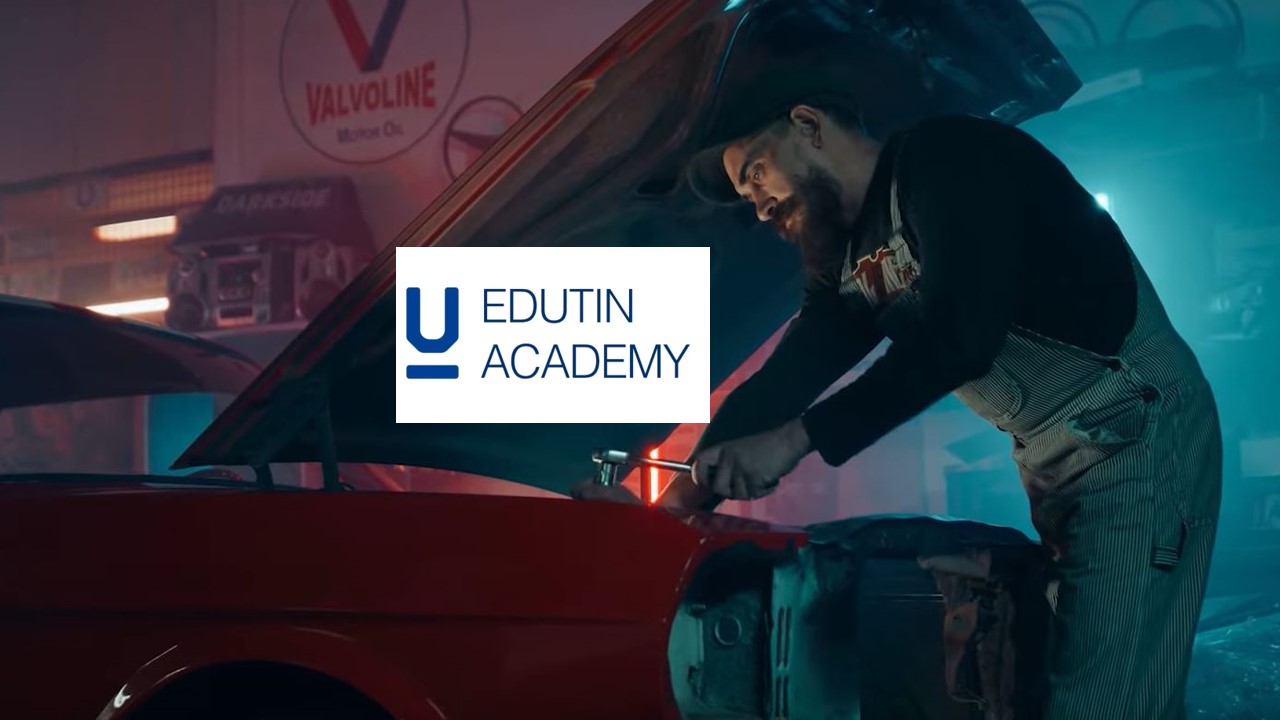
Curso de Mecánica Automotriz: ¡Gana $40,000 al año!
¡Puedes realizar un curso completo de Mecánica Automotriz gratis directamente a través de la plataforma de Edutin! ¡Mira cómo funciona!
Continúe Leyendo